Yield prediction and forecasting models are transforming the way agronomists, farmers, and agritech firms manage crop output in the quickly changing agricultural world of today.Yield Prediction and Forecasting Models- Accurate yield prediction is now essential for sustainable food production and effective resource management as climatic unpredictability, rising global demand, and environmental issues continue to worsen. We go deeply into the realm of yield prediction and forecasting models in this blog article, examine the newest technology that powers these models, and talk about how they might improve agricultural operations.
Overview
Estimating the quantity of crop that will be produced per unit area prior to harvest is known as yield prediction. To produce these forecasts, forecasting models use sophisticated statistical techniques, real-time inputs, and historical data. These models may provide priceless insights that support farmers in making prompt and well-informed choices by using data from weather stations, soil sensors, remote sensing, and machine learning algorithms.
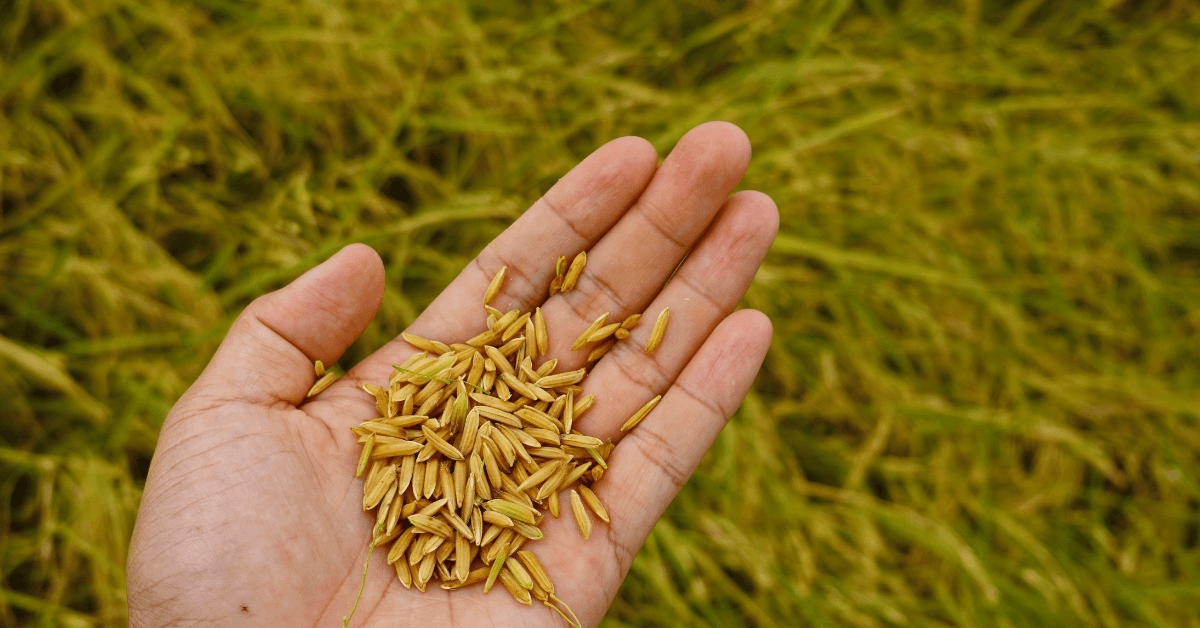
Precise yield projections are essential for:
We are allocating resources (labor, fertilizer, and water) as efficiently as possible.
Improving market pricing forecasts and supply chain planning
Precision agriculture’s ability to lessen environmental consequences
Increasing the overall sustainability and profitability of farms
The development of yield forecasting-Yield Prediction and Forecasting Models
In the past, farmers used crude statistical techniques, weather measurements, and firsthand experience to forecast crop yields. However, the intricacies of contemporary agriculture, such as soil variability and climate change, were often beyond the scope of conventional methods. With the advent of data analytics and artificial intelligence, yield prediction has entered a new phase where enormous volumes of data are used to provide accurate and useful predictions.
Important Factors in Contemporary Yield Forecasting
Big Data and IoT: As the Internet of Things (IoT) has grown in popularity in agriculture, sensors that continually check plant health, temperature, humidity, and soil moisture have been widely installed. The accuracy of yield prediction models is increased by feeding them this real-time data.
The Technologies Underpinning Forecasting and Yield Prediction Models-Yield Prediction and Forecasting Models
Technologies for Gathering Data
A combination of many data streams is used in modern yield prediction:
1. Soil sensors:
Keep an eye on the moisture content, pH, and nutrient levels of the soil.
2. Meteorological stations:
Offer current and historical meteorological information, including humidity, precipitation, and temperature.
3. Drones and satellite imagery:
Take high-resolution photos that are then processed to calculate vegetation indices, such as the Normalized Difference Vegetation Index (NDVI), which has a significant relationship with plant health.
IoT devices: Provide a detailed picture of environmental conditions by continuously gathering data from fields.
Analytics and Data Processing-Yield Prediction and Forecasting Models
Following collection, a variety of analytical methods are used to process the data:
1. Statistical Modeling:
Historical yields and environmental factors have been correlated using conventional regression models.
Machine learning algorithms are capable of handling complicated interactions and non-linear correlations between variables. Examples of these algorithms include random forests, gradient boosting machines, and neural networks.
2. Deep Learning:
To make more precise yield forecasts, convolutional neural networks (CNNs) and recurrent neural networks (RNNs) are being employed more and more to evaluate picture data and temporal sequences.
Platforms for Integration
It is essential to integrate various datasets. Specialized software programs and cloud-based platforms enable stakeholders to:
Maintain and store vast amounts of data.
Execute intricate models that use historical yield records, satellite photos, and real-time sensor data.
Make forecasts come to life using dashboards that show important patterns and irregularities.
Advantages of Precise Yield Forecasting-Yield Prediction and Forecasting Models
There are many advantages to accurate yield forecasting for different parties involved in the agricultural value chain:
Regarding Farmers:
Improved Resource Management:
When farmers have accurate production forecasts, they can more effectively schedule the use of herbicides, fertilizers, and irrigation. As a result, waste is minimized, and resources are utilized more efficiently, ultimately leading to cost savings and environmental benefits.
Better Crop Management:
Similarly, by identifying potential production decreases due to insect outbreaks or unfavorable weather conditions early, farmers can take immediate remedial action. Consequently, this proactive approach helps mitigate losses and maintain crop health.
Increased Profitability:
Furthermore, predictive insights enable better planning for planting, harvesting, and storage. As a result, farmers can reduce costs, optimize operations, and ultimately boost profitability.
For supply chains and agribusiness
Market Price Forecasting:
Businesses can estimate supply levels with the use of accurate yield data, which lowers the danger of shortages or surpluses and stabilizes market prices.
Effective Distribution and Logistics:
Supply chain managers may minimize transportation expenses and guarantee that food reaches markets on schedule by optimizing logistics with the help of accurate yield projections.
Strategic Investment Decisions:
To match supply with demand, agribusinesses may utilize yield forecasts to direct investments in new technologies and growth initiatives.
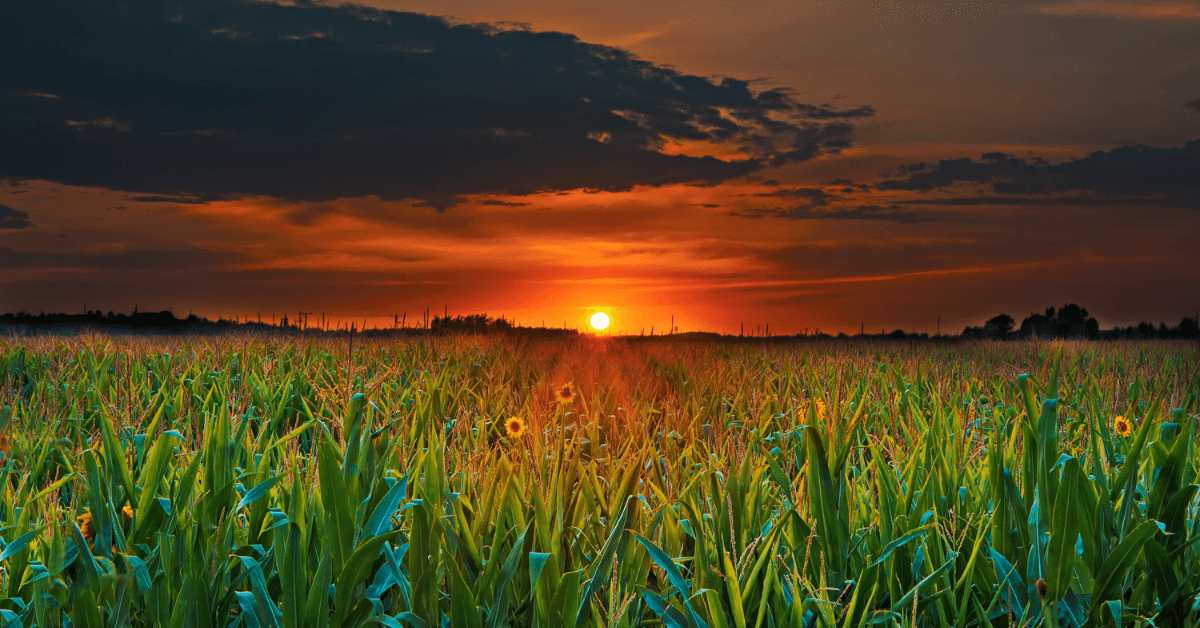
For researchers and policymakers.
Food Security Planning:
To guarantee that people have consistent access to wholesome food, national and international organizations may use yield projections to create food security plans.
Climate Adaptation Strategies:
By combining yield projections with climate models, it is possible to pinpoint areas that are at risk and create focused plans to lessen the effects of climate change on agriculture.
Sustainable Agriculture:
By lowering input usage and limiting the environmental impact of agricultural operations, yield prediction models support sustainable farming methods.
Yield Prediction Difficulties
Notwithstanding the many advantages, there are still a number of difficulties in the field of yield forecasting and prediction:
Integrity and Quality of Data
Heterogeneous Data Sources:
It may be challenging to combine data from several sources, such as historical documents, satellite photography, and ground sensors. It is essential to guarantee data correctness and consistency.
Sensor failures or gaps in past data can jeopardize the accuracy of the model. To solve these problems, strong data cleaning and imputation techniques are required.
Interpretability and Complexity of the Model
Overfitting:
When advanced models—especially deep learning systems—overfit to training data, they may perform poorly in novel circumstances.
Interpretability:
Complex models may act as “black boxes,” making it difficult for stakeholders to comprehend the underlying decision-making process, even though they often provide very accurate results. It is crucial to strike a balance between interpretability and accuracy.
Variability in the environment
Climate Change:
Yield projections may become questionable due to erratic weather patterns and climatic abnormalities. Models have to constantly adjust to fresh climatic data and changing circumstances.
Local Variability:
Even within the same field, microclimates and soil characteristics may differ greatly. Localized models and high-resolution data are required to properly reflect this variability.
Operational and Economic Limitations
Adoption Obstacles:
A lack of digital literacy and infrastructure issues in certain areas can hinder the use of yield prediction tools.
Prospective developments in yield forecasting and prediction
Yield prediction has several exciting aspects and is growing quickly:
Using advanced AI and machine learning
Enhanced Capabilities for Remote Sensing
Higher Resolution Photography:
More accurate crop health and growth monitoring will be possible with the availability of higher resolution satellite and drone photography.
By providing comprehensive data on plant stress, nutritional status, and disease incidence, multispectral and hyperspectral imaging technologies help to improve production forecasts.
A rise in the use of cloud-based platforms
Collaboration and Data Sharing:
Cloud systems make it easier for stakeholders and geographical areas to share agricultural data, which promotes teamwork and increases model robustness.
Scalability:
Cloud computing enables models to scale across wide geographic regions, providing insights at both local and national levels.
Combining Sustainable Agricultural Methods
Precision agriculture:
We will gradually incorporate yield prediction models into precision agricultural systems to enable focused input application and reduce environmental effects.
Sustainable Resource Management:
In order to help efforts for sustainable agriculture, future models will not only forecast production but also provide suggestions for maximizing resource usage.
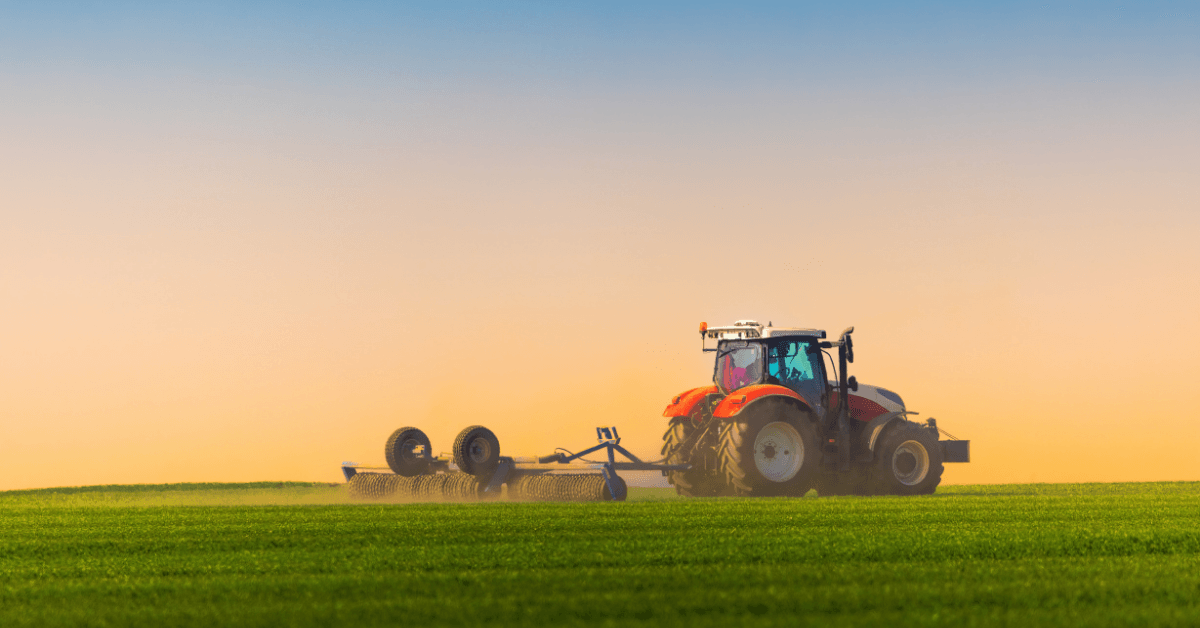
Success Stories and Case Studies
Corn and Soybean Production in the United States
Yield models play a crucial role in managing the enormous US maize and soybean crops. By integrating satellite images, real-time weather data, and historical yield records, researchers have developed highly accurate models, often precise to just a few bushels per acre. As a result, this improved accuracy enables farmers to optimize their operations more effectively, ultimately maximizing both yield and profit.
Asian Rice-Fish-Duck Systems
Africa’s Precision Farming
African smallholder farmers are utilizing smartphone yield prediction apps that monitor weather and soil conditions using machine learning algorithms. These technologies allow climate-vulnerable farmers to vary planting times and input amounts, improving output and food security.
How Your Farm Can Use Yield Prediction
Here are some concrete actions that farmers and agribusinesses may take to incorporate yield prediction algorithms into their operations:
To collect real-time field data, invest in sensors, weather stations, and drones or satellites.
Utilize powerful analytics, storage, and data integration cloud services. Simple visualization and dashboards are common in these systems.
Agritech supplier collaboration:
Work with yield prediction model designers and implementers. Their experience can help you tailor models to crops and regions.
Educate and train your staff:
Ensure you and your farm management team are familiar with the new technology. Successful deployment requires training on analyzing data and adjusting management practices as needed.
Yield prediction models require continuous monitoring and improvement. Regularly track the model’s performance and make adjustments as conditions and data change.
In conclusion
Agriculture is quickly changing with yield prediction and forecasting. Using machine learning, remote sensing, and advanced data analytics, these models help farmers and agribusinesses maximize resource usage, crop management, and food security, even under changing circumstances.
Despite data integration, rudimentary models, and environmental uncertainty, exact yield forecasting has clear benefits. These strategies will become increasingly more important for sustainable farming as technology advances.
Using agricultural yield forecasting techniques is essential for industry leadership. These technologies will also help multinational firms and smallholder farmers compete globally.
An Appeal for Action
Are you ready to fully utilize forecasting and yield prediction models on your farm or business? For the most recent information, technology advancements, and professional advice on precision agriculture, sign up for our newsletter. Take the first step toward using cutting-edge data analytics to revolutionize your agriculture operations by joining our community now. Keep yourself updated, maintain your edge, and contribute to the development of sustainable agriculture!
FAQ:
What is yield prediction?
- Historical data, climate patterns, and remote sensing collectively help predict agricultural yield. Moreover, farmers leverage advanced analytics and machine learning to make informed decisions about resource allocation. As a result, they can optimize inputs such as fertilizers, water, and pesticides, ultimately improving efficiency and productivity.
How do you predict yields?
- Historical, weather, soil, and crop sensors predict yields. Modern algorithms and machine learning models predict yields using these traits.
How do you estimate crop yield?
- Reliable agricultural production estimates come from past data, remote sensing images, and plant biomass and density assessments. From this data, statistical models estimate yield.
What are the models of yield estimation?
- Many yield prediction systems use regression analysis, random forests, and neural networks to analyze soil, weather, and remote sensing data.
What is the model for crop yield prediction?
- A typical agricultural yield forecast model uses advanced machine learning algorithms and incorporates weather, remote sensing, and past yield data.