Overview
Agriculture is changing because of new technologies. This is a business that has been around for thousands of years. Machine Learning for Yield Prediction- Farmers can now use data to make better and smarter decisions about crop management, thanks to the addition of machine learning for crop yield prediction. This change should make farms more efficient, last longer, and make more money. But how does machine learning help with predicting yields, and what can farmers expect from this technology?
We are using machine learning (ML) to predict crop yields. This article will discuss the technologies used, their main benefits to farmers, and how they could change farming. We will also discuss the challenges that arise when implementing ML in farming and provide guidance on how to initiate the process.
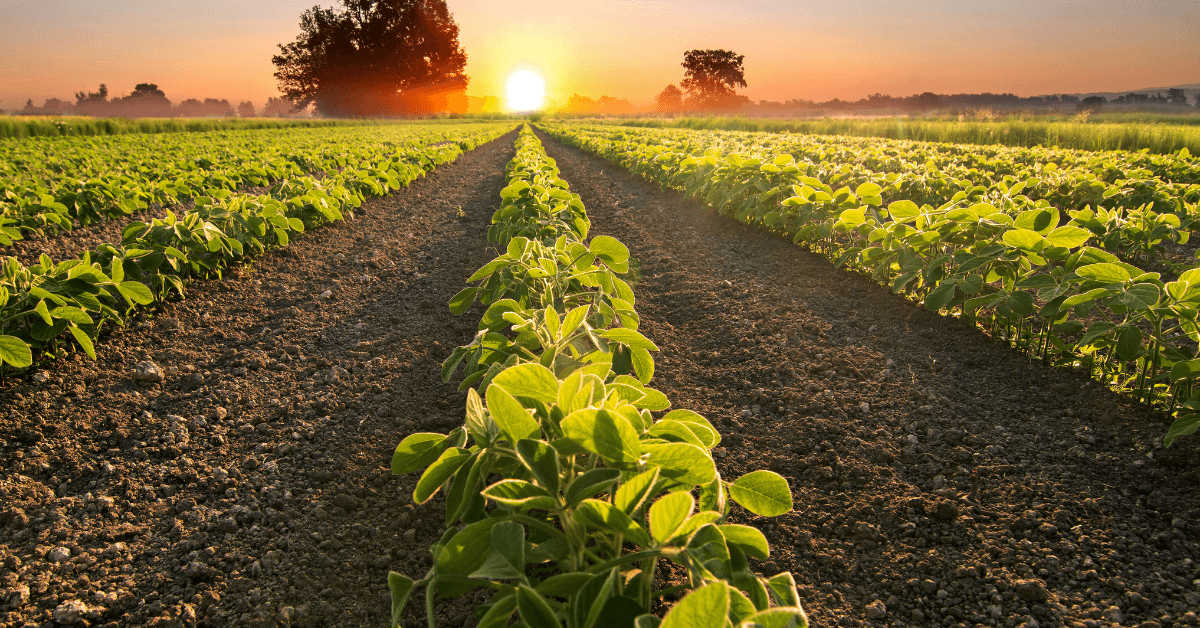
How can we understand machine learning in farming?-Machine Learning for Yield Prediction
Artificial intelligence (AI)’s machine learning branch enables computers to learn from data and improve over time without human intervention. In farming, machine learning systems look at a lot of data from different places, like satellite images, weather trends, soil conditions, and the health of crops. We then use these observations to predict crop yield and enhance farm efficiency.
Machine learning has a lot of promise in agriculture. It can help farmers cut down on risks and get more done by making accurate guesses based on past data. ML systems can guess how crops will do in a certain season by looking at past data, weather forecasts, the health of the land, steps taken to keep pests away, and a lot more.
How Data Can Help You Guess Yield-Machine Learning for Yield Prediction
Data is what machine learning is all about when it comes to predicting results. To create effective prediction models, we require high-quality data. Different facts play a crucial role in estimating crop quantity.
- Weather Information: Things like weather, rainfall, and humidity in the air can have a direct effect on food growth. Machine learning systems use this information to predict how different weather conditions will affect the food yields of specific crops.
- Data on the earth: The pH, fertilizer levels, and moisture content of the soil can have a big effect on how well crops grow. Machine learning models use this information to make more accurate predictions about the growth of certain crops in different types of soil.
- Satellite and Drone Images: Pictures taken by satellites and drones show how crops are doing, if there are pests, and how the weather is affecting them in real time. Machine learning systems can look at these pictures to find problems early on and guess how they will affect crop growth.
- Historical Yield Data: We build machine learning models based on historical yield data. Algorithms can find trends and patterns that are important for predicting future harvests by looking at data from previous seasons.
- Remote Sensing Data: Tools for remote sensing, such as weather stations and satellite monitors, continuously monitor the farming area. Real-time yield prediction requires this information.
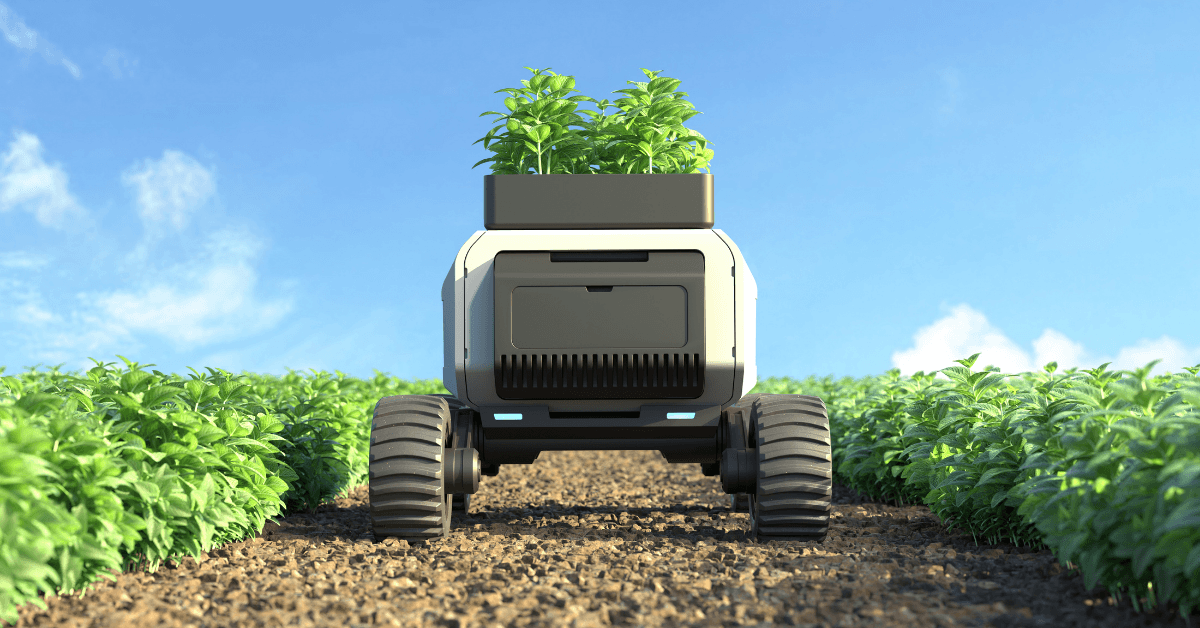
What does machine learning do to predict yield?
There are several steps involved in using machine learning to estimate food yields.
- Collecting Data: The first step is to get data from a number of different sources. This includes information about the quality of the land, the weather, pests, the health of the crops, and past results.
- Process Data: You must clean up and preprocess raw data before using it for models. In this step, you get rid of noise, deal with missing numbers, and normalize the data so that machine learning programs can use it.
- Teach the model: The next step involves using the prepared dataset to teach machine learning techniques. Decision trees, random forests, and neural networks are some of the most common methods used to make models that can use past data to guess what future returns will be.
- Prediction: After training, the model predicts the crop amounts for the upcoming season. With new data, the model learns and gets more accurate over time. We regularly update it with new data on crop health, weather trends, and soil conditions.
- Validation and Fine-Tuning: We check the model’s predictions against real results to determine their accuracy. We examine any discrepancies between the expected and actual results, and refine the model to enhance its performance.
Using machine learning to predict yield is a good idea-Machine Learning for Yield Prediction
Farms benefit from using machine learning to predict crop yields in a variety of ways.
- Yield Predictions That Are More Accurate: One of the best things about machine learning is that it can predict crops more accurately than standard methods. Machine learning algorithms find trends that would be impossible for people to see by looking at huge amounts of data. This makes predictions more accurate
- Better use of resources: Farmers can make the best use of resources like water, chemicals, and labor when they have correct predictions of crop yields. Knowing when and where to use these resources can help you cut down on waste, save money, and get better crops.
- Better crop management: Machine learning gives farmers better crop management information by showing them when to plant, water, and gather their crops. By forecasting their crop yields, farmers can make smart decisions to maximize yield.
- Reducing risk: Farmers can get ready for adverse weather by figuring out what kinds of yield fails or losses might happen. For example, machine learning models can let farmers know about droughts or pest problems in advance, giving them time to take steps to protect their crops.
- Sustainability: Farmers can use lessons from data to make their farming methods more environmentally friendly. Machine learning helps people use less water and chemicals than they need to. In the long run, this is good for the earth, land, and ecosystem.
There are issues with implementing machine learning in farming
Despite the great potential of machine learning, implementing it effectively can be challenging for several reasons:
- Quality and Quantity of Data: The effectiveness of machine learning models rests on how much and what kind of data is available. Farmers in some areas might not have access to enough data or data sources they can trust, which could make ML models less useful.
- High start-up costs: The use of machine learning in farming requires a significant investment in equipment such as sensors, drones, satellites, and data processing systems. This can be challenging for small farmers, but over time, prices should decrease.
- Not enough technical know-how: Machine learning needs knowledge of AI and data science, which many farms may not have had official training in. This lack of understanding can make it challenging to accept and use machine learning correctly.
- Integration with Current Systems: Many farms currently utilize outdated tools and methods to monitor results. It can be challenging and take a lot of time to connect machine learning models to current systems and processes.
- Changes in the climate: Even though technology has come a long way, it is still difficult to say for sure what food yields will be because the weather can change quickly and in ways that affect plant growth.
What the future holds for machine learning in farming
As technology keeps getting better, machine learning in agriculture seems like it will have a bright future. Observe the following trends and developments:
- Better data collection: Better satellite photos, drones, and cameras will capture more comprehensive and real-time data to improve machine learning models.
- More farm equipment will employ AI and machine learning for planting, watering, and harvesting. This optimizes resources and boosts agricultural production.
- Machine learning algorithms can predict when farming equipment would break down, saving money on repairs and improving operations.
- Blockchain for Data Integrity: As data collection and sharing become more important, blockchain technology might provide data integrity and transparency, allowing farmers to trust machine learning model forecasts.
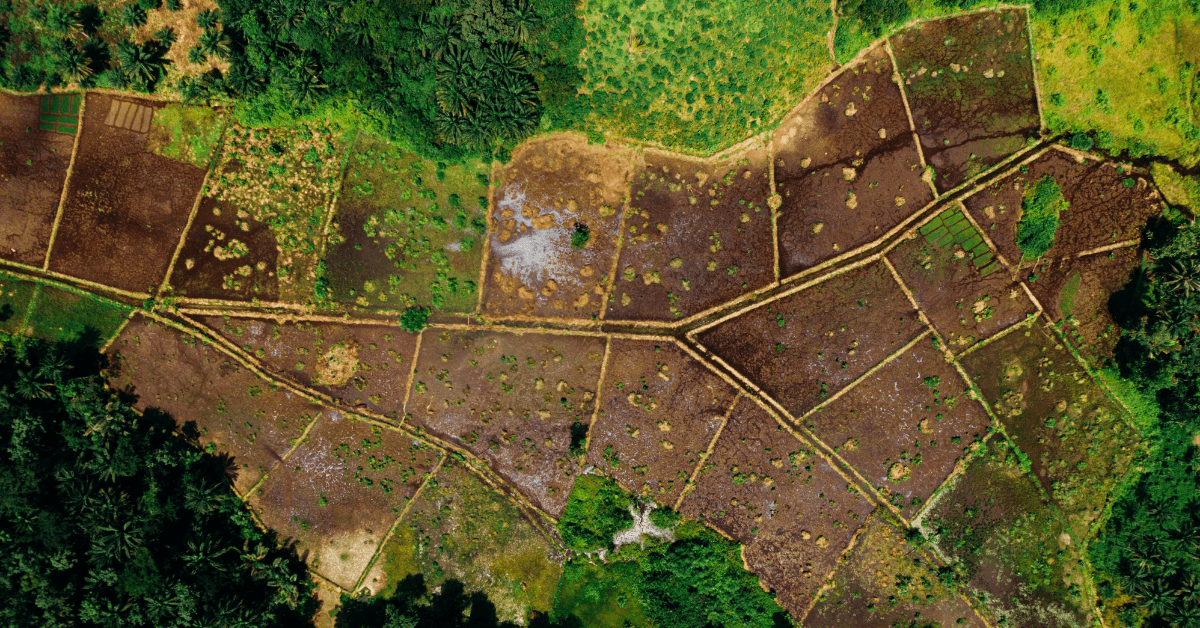
How to Start Using Machine Learning to Guess Yield
If you are a farmer who wants to use machine learning to guess crop yields, here are the steps you need to take:
- Get useful information: To start, get information from a number of different places, such as weather reports, information about the land, and past crop yields. Many farmers already obtain some of this information manually or through various devices.
- Spend money on tools and software. Machine learning-driven tools for yield prediction are available on several software platforms. Some of these platforms are designed for farming and have simple tools to help farmers get started.
- Work with experts. If you don’t know much about machine learning, you might want to work with a data scientist or a farming technology expert who can help you put the models into action and figure out what they mean.
- Begin small and grow: To test the method, start with a small part of your farm. You can use machine learning in more parts of your business once you’re pleased with the results.
- Stay current: To maximize this powerful tool, stay current on AI and machine learning technologies and trends.
In conclusion, welcome the future of farming
Machine learning for predicting crop yields is not just a passing fad; it is a force that is changing the farming business. By using data and AI, farmers can make better decisions, utilize resources more efficiently, and produce more crops with less environmental impact. While there are some challenges, the advantages greatly surpass the drawbacks, and with the ongoing advancements in technology, machine learning could find its application in any farming scenario.
Are you prepared to enhance the productivity and durability of your farm? It’s time to accept the future with machine learning. Start looking into the options right away!
What You Should Do:
Are you interested in utilizing machine learning to forecast crop growth on your farm? Get in contact with us to find out how we can help you use AI-powered tools to handle your crops better!
FAQ:
What is the definition of yield prediction machine learning?
- Using statistical models and algorithms, machine learning for yield prediction analyzes several data sources, including weather, soil health, and past yields, to forecast crop yields. Farmers can improve their decision-making and resource optimization by utilizing machine learning models that can accurately predict future crop yield, utilizing both historical and real-time data.
How do crop yield predictions come from machine learning?
- By examining vast datasets from several sources, including soil conditions, meteorological data, and satellite photos, machine learning forecasts agricultural yields. We process this information using algorithms to identify trends and connections among the variables that impact crop growth. Once trained, the algorithm can forecast future yields, taking into account factors such as soil changes and weather.
What advantages does using machine learning to yield predictions offer?
- Using machine learning to estimate yield has several advantages, such as improved crop management, improved accuracy, and better resource management. Accurate production projections help farmers maximize crop quality and minimize waste by optimizing water use, fertilizer application, and harvesting dates. Furthermore, machine learning reduces risks by forecasting unfavorable circumstances like insect outbreaks or droughts.
What information is required to use machine learning to estimate yield?
- Machine learning algorithms need information on past yields, weather trends, soil quality, and crop health in order to make reliable agricultural yield predictions. Additionally important are sensor-based data (such as moisture levels) and remote sensing data from satellites or drones. Algorithms may use these datasets to find relationships and forecast results for different crop varieties under varied circumstances.
Is it possible to train various crops using machine learning?
- It is possible to forecast yields for a variety of crop types using machine learning. We can customize models to individual crops by incorporating pertinent data on the crop’s growth cycle, environmental parameters, and geographical circumstances. Precision agriculture uses machine learning, a flexible method, to optimize yield estimates for a variety of crops, including vegetables and cereals.