Integrated AI Systems: Transforming Technology via Smooth Cooperation
Although businesses have changed as a result of artificial intelligence (AI), complicated challenges are frequently beyond the scope of standalone AI technologies. Integrated AI Systems-Let’s introduce integrated AI systems, which are sophisticated networks that combine multiple AI technologies to deliver solutions that are more intelligent, efficient, and versatile. We’ll look at the advantages, difficulties, and practical uses of these systems in this blog. Let’s get started!
Integrated AI Systems: What Are They?-Integrated AI Systems
Integrated AI systems combine robotics, computer vision, natural language processing (NLP), and machine learning into a single framework. These systems, in contrast to isolated models, exchange data, learn from one another, and adjust to changing conditions. A self-driving car, for instance, processes sensor data, detects pedestrians, and navigates traffic all at once using integrated AI.
Through the integration of many technologies, these systems simulate human decision-making. They provide smooth communication between components by dismantling data silos. As a consequence, companies’ operations become more accurate, efficient, and scalable.
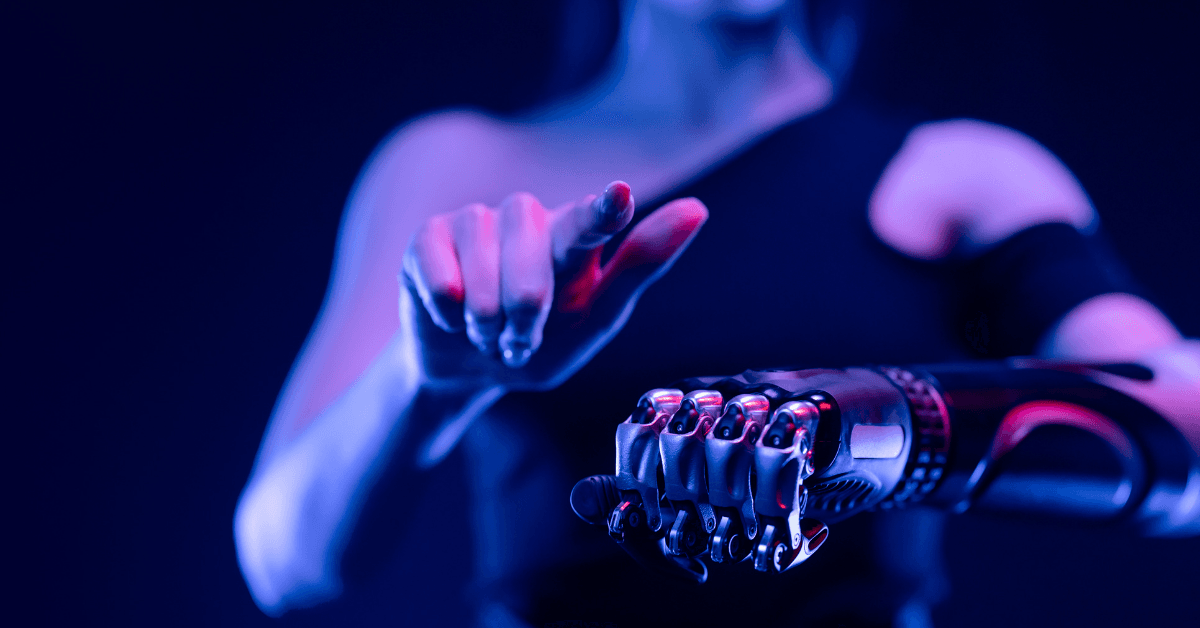
1. Better cooperation leads to better decision-making with integrated AI systems.
Real-time data analysis from many sources is possible with integrated systems. For example, a healthcare AI uses imaging scans, test findings, and patient history to detect illnesses more quickly. Data cross-referencing lowers mistakes and reveals trends that single models would overlook.
2. Enhanced effectiveness and allocation of resources-Integrated AI Systems
When AI technologies work together, they simplify workflow automation. Supply chain managers employ integrated technologies to automatically manage inventories, optimize routes, and forecast demand. This method reduces the need for human involvement and lowers operating expenses
3. The capacacross scale Across sectors
Integrated AI adjusts to industry-specific requirements in anything from retail to agriculture. Farmers use these technologies to automate irrigation, analyze soil data, and use drones to check crop health. This adaptability guarantees broad applicability.
4. Quicker Resolution of Issues
When AI modules collaborate, they can quickly address complex problems. These days, banking fraud detection systems use machine learning and natural language processing (NLP) to identify suspicious transactions immediately.
Essential elements of integrated AI frameworks-Integrated AI Systems
Learning Machines (ML) ML algorithms are the foundation of integrated AI. Neural networks and decision trees are examples of algorithms that use data to learn and forecast results. Through feedback loops, they improve their accuracy over time.
Processing Natural Language (NLP)
Systems can comprehend and produce human language thanks to NLP. For instance, chatbots evaluate consumer inquiries and offer pertinent answers using natural language processing (NLP).
Vision in Computers
Machines can now “see” and comprehend visual data thanks to this technology. When combined with machine learning, it powers driverless cars, facial recognition, and industrial quality control.
Integration of Robotics and IoT
AI-driven choices are carried out by physical devices such as IoT sensors and robotics. Smart factories use robots to assemble goods, while IoT sensors monitor the condition of the machinery.
Infrastructure for Cloud Computing
The processing power required for extensive AI operations is supplied by cloud platforms. They also make it easier for modules to collaborate in real time and save data.
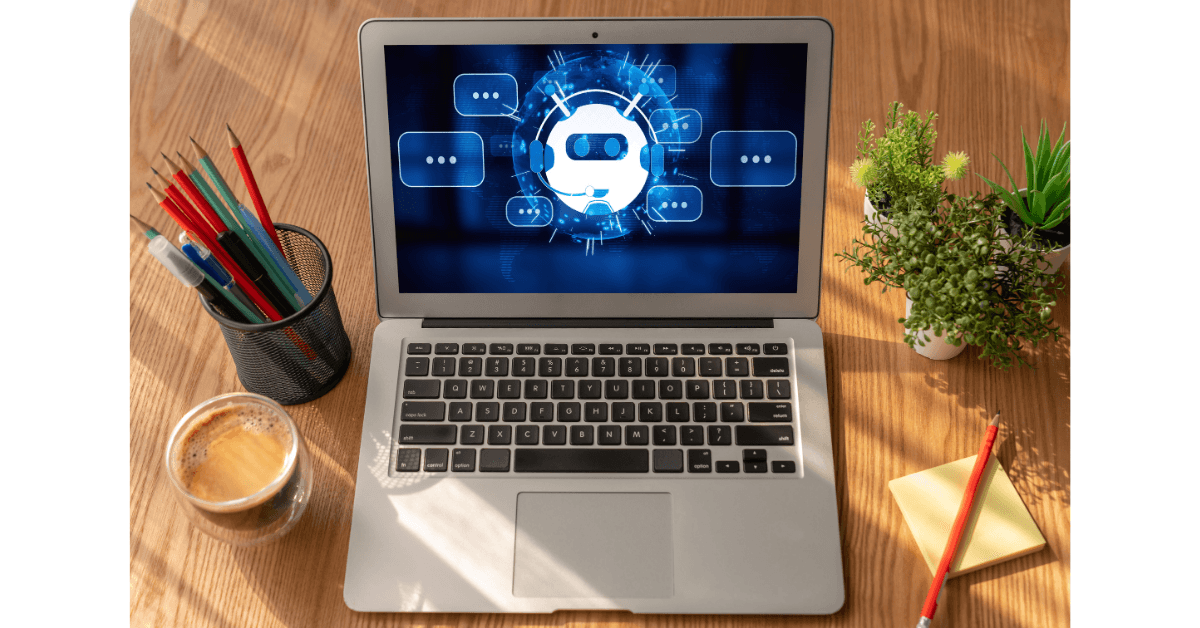
Difficulties in developing integrated AI systems
1. Problems with Data Compatibility
Various AI technologies frequently call for data in different formats. For example, combining unstructured social media feeds with structured sales data necessitates a lot of preparation.
2. Expensive Development
Proficiency in several AI fields is necessary to create interconnected systems. Businesses need to make investments in strong infrastructure, high-quality datasets, and qualified engineers.
3. Privacy and Ethical Issues
Data centralization raises the possibility of misuse. To adhere to laws such as the GDPR, developers must incorporate privacy protections, such as anonymizing user data.
4. Maintenance Complexity
An update to one module may impact other modules. To guarantee seamless functioning, regular testing and version control are crucial.
Applications of Integrated AI in Healthcare in the Real World: Precision Medicine-Integrated AI Systems
Hospitals use AI to customize care. To suggest customized treatments, systems examine clinical trials, lifestyle variables, and genetic data. IBM Watson Oncology, for instance, makes recommendations for cancer therapy based on information unique to each patient.
Retail: Extremely Tailored Purchasing
NLP, ML, and computer vision are all used in e-commerce systems to improve customer experience. Amazon’s recommendation engine makes product recommendations based on browsing and purchasing history.
Manufacturing: Predictive maintenance facilities use IoT sensors and machine learning to anticipate machine breakdowns. Siemens employs these devices to notify technicians of turbine problems prior to malfunctions.
Transportation: Self-Driving Cars
To allow self-driving cars, companies such as Tesla use machine learning, sensor data, and computer vision. To protect the safety of passengers, these technologies process real-time traffic information.
Finance: Identifying Fraud
Banks use integrated AI to monitor transactions. While ML algorithms identify odd spending patterns, NLP checks consumer emails for phishing efforts.
Integrated AI Systems’ Future
1. Using Edge AI to Lower Latency
Instead of depending entirely on the cloud, future systems will use edge computing to process data locally. This technique reduces delays in vital applications, such as drones for emergency response.
2. Using low-code platforms to democraticize
Non-experts will be able to create integrated AI systems with low-code tools. Drag-and-drop interfaces may enable small firms to automate processes without requiring coding knowledge.
3. Frameworks for Ethical AI
Transparency will be a top priority for developers as rules tighten. By elucidating how computers make judgments, explainable AI (XAI) will increase user confidence.
4. Integration of Quantum Computing
AI with quantum capabilities might resolve challenging issues in a matter of seconds. Researchers are investigating its potential for climate prediction and medicine development.
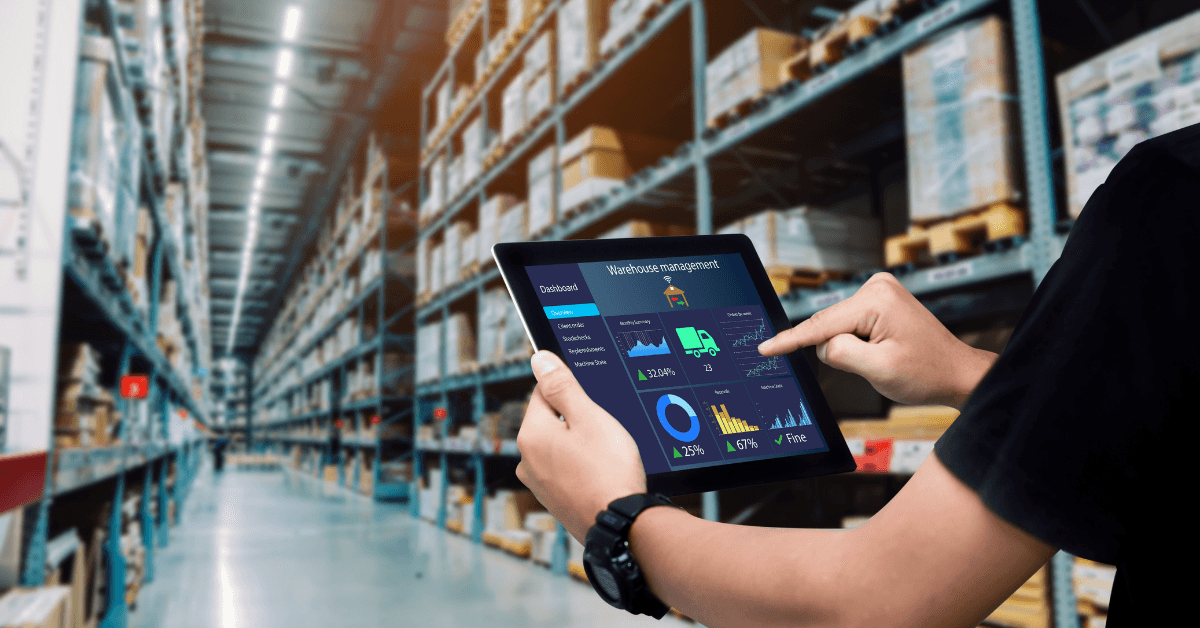
How to Successfully Use Integrated AI: Start Small
Start with a pilot project, such as using chatbots to automate customer support. Scale slowly as you learn more.
Make an investment in data quality.
Data that is clean and tagged is essential. To efficiently arrange various datasets, use techniques such as data lakes.
Encourage interdisciplinary teams.
Work together with domain experts, engineers, and data scientists. Having a variety of viewpoints helps avoid blind spots.
Put Security First
Perform routine audits and encrypt critical information. Keep abreast of the criteria for compliance.
In conclusion, embrace the revolution in integrated AI.
Because they provide previously unheard-of speed, precision, and flexibility, integrated AI systems are revolutionizing problem-solving. While issues like ethics and data complexity still exist, developments in edge computing and quantum AI hold up the possibility of fascinating new discoveries. Early adopters of these solutions will be at the forefront of customer happiness, efficiency, and innovation.
Are you prepared to use AI in your business? Please identify a problem initially, then assemble a knowledgeable team and utilize scalable cloud solutions. AI’s future lies in their smooth cooperation, not merely in autonomous tools.
FAQ:
What is integrative AI?
- By combining many AI technologies, integrative AI makes cooperative problem-solving possible. Systems increase efficiency by sharing workflows and data. They improve real-time judgments in sectors like healthcare and finance by adapting dynamically.
What are the 4 types of AI systems?
- Without memory, reactive machines react to stimuli. Restricted Memory AI makes choices based on historical data. The theory of mind understands emotions. Theoretically, self-aware artificial intelligence is both conscious and self-aware.
How to integrate AI in a system?
- Please begin by establishing objectives and data sources. Next, pick appropriate AI technologies such as NLP or ML. Then, for connection, utilize APIs. Lastly, optimize performance through iterative testing.
What does Integrate AI do?
- Robotics, machine learning, and natural language processing are all part of integrated AI. It finds insights, automates activities, and optimizes workflows. Companies utilize it to improve user experiences, scalability, and accuracy.
How can AI be integrated?
- Please ensure that AI is aligned with business requirements as a priority. For scalability, use cloud platforms. Use APIs to link modules. Use high-quality data to train models. Track results and make constant process improvements.