How AI Enhances Manufacturing Quality Control
The manufacturing sector depends on accuracy, productivity, and reliability. Even small flaws can result in costly recalls, tarnished reputations, or safety risks. How AI Improves Quality Control in Manufacturing-Despite their effectiveness, traditional quality control techniques frequently fall behind the needs of contemporary manufacturing. Artificial intelligence (AI) is a transformative technology that is revolutionizing the way producers identify defects, streamline operations, and produce flawless goods. Let’s investigate how AI raises the bar for quality control.
1. Computer Vision-Based Real-Time Defect Detection
Computer vision systems driven by AI are now faster and more accurate than human inspectors. These devices, which are outfitted with deep learning algorithms and high-resolution sensors, evaluate items on assembly lines in milliseconds. AI-powered semiconductor factories, for instance, are able to examine hundreds of microchips per hour and identify minute fractures or misalignments that are undetectable to the human eye.
The use of AI instead of manual inspections reduces human fatigue errors. Employees no longer have to spend hours staring at conveyor belts. Rather, they concentrate on resolving issues that have been recognized, increasing productivity. Additionally, AI uses continual learning to adjust to new fault patterns. Without needing reprogramming, the system modifies its detection criteria in the event that a new kind of scratch appears.
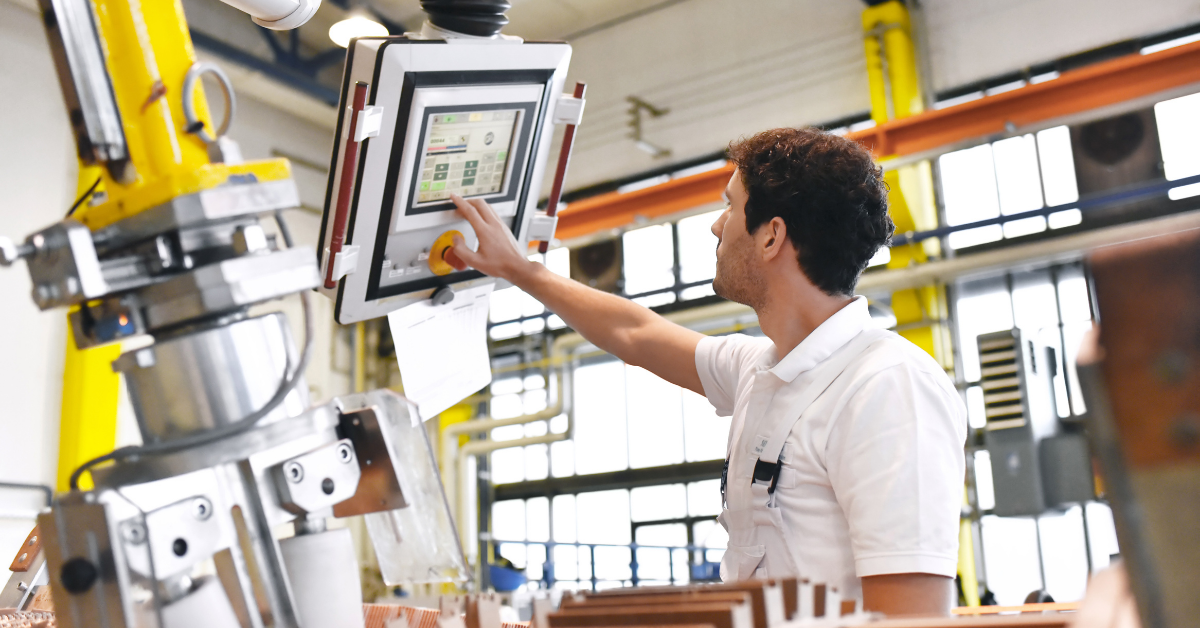
2. Preventing Equipment Failures with Predictive Maintenance-How AI Improves Quality Control in Manufacturing
Production is disrupted, and product quality is compromised by machinery failures. AI addresses such issues by anticipating malfunctions before they happen. Sensors gather information from devices such as robotic arms or CNC machines about temperature, vibration, and energy usage. Machine learning algorithms then detect anomalies indicating wear and tear.
For instance, an automobile facility may use AI to supervise welding robots. When motor vibrations surpass safe limits, the system notifies professionals, allowing for prompt repairs. This proactive strategy guarantees continuous output quality and reduces unscheduled downtime. Additionally, predictive maintenance prolongs the life of machinery, saving manufacturers millions of dollars in replacement expenses.
3. Complex product-automated inspection systems
Strict inspections are necessary for complex items like medical gadgets or aeronautical components. Conventional techniques sometimes include laborious hand measurements or damaging testing. AI-powered automated inspection technologies streamline this procedure.
Consider the turbine blades found in aircraft engines. AI compares each blade to digital twins using generative adversarial networks (GANs) and 3D scanning. Deviations as small as 0.01mm trigger instant notifications. This degree of accuracy reduces inspection time by up to 70% while guaranteeing adherence to stringent safety regulations.
4. Using Data Analytics to Improve processes-How AI Improves Quality Control in Manufacturing
AI detects flaws and stops them. AI finds the underlying reasons for quality problems by examining both historical and current production data. Let’s say a beverage manufacturer observes irregular amounts of bottle fill. To identify the offender, AI compares data such as conveyor speed, temperature, and valve pressure.
AI also suggests the best settings to preserve quality. The device automatically modifies the curing oven settings if humidity impacts the adhesive strength during smartphone assembly. Manufacturers may increase yield rates, cut waste, and improve operations with the help of these insights.
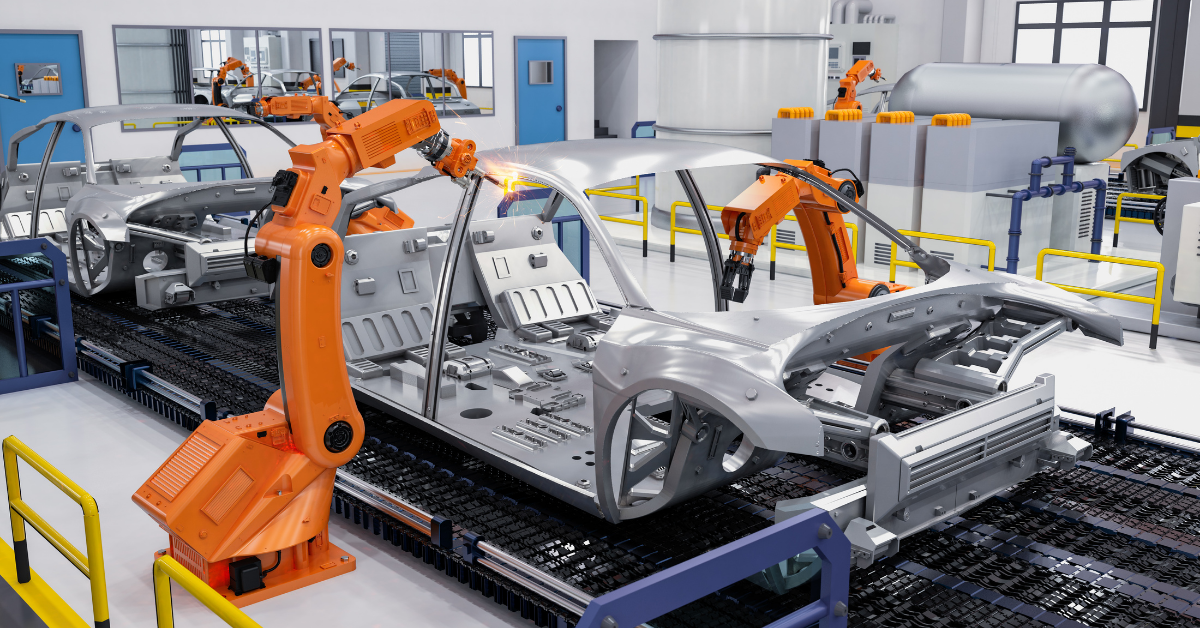
5. Improving Quality Control in the Supply Chain
Long before goods are put on the manufacturing line, quality control is implemented. AI monitors suppliers’ raw materials and components to ensure compliance with requirements. Tools for natural language processing (NLP) look for inconsistencies in supplier certificates and documentation.
For instance, a food company might use AI to assess the quality of grain in shipments. By identifying impurities like mold or pesticides, hyperspectral imaging can quickly reject inferior batches. This upstream vigilance safeguards the integrity of the final product by keeping defective materials out of the manufacturing process.
6. Improving Consistency and Reducing Human errors
Despite their experience, human inspectors are prone to attentional errors. AI systems, on the other hand, are constantly focused. AI checks fabric rolls for color irregularities or weaving flaws in the textile production process with 0% variability.
Employees also gain. They upskill to positions like data analysis or AI system monitoring in place of repetitious duties. This change promotes a continual improvement culture in addition to increasing work satisfaction.
7. Cost Cutting and ROI Improvement-How AI Improves Quality Control in Manufacturing
AI quality control implementation yields quick results. Early fault discovery reduces rework expenses and scrap rates. According to Deloitte research, AI may save up to 25% on quality-related costs in the first year.
AI also reduces the expenses associated with warranty claims and recalls. Manufacturers safeguard the reputation of their brands and stay out of trouble with the law by making sure that only perfect items are delivered to consumers. These savings eventually balance the initial AI investment, producing an attractive return on investment.
8. Upcoming Trends: Integration of AI and IoT
Combining AI with the Internet of Things (IoT) is the way of the future for quality control. Production lines are equipped with networked sensors in smart factories, which provide AI platforms with real-time data. End-to-end visibility is made possible by this synergy.
Consider a smart electronics manufacturing facility where AI monitors every step of the process, from soldering to packing. IoT sensors identify the problem if a soldering robot breaks down, and AI moves batches to backup units. Even in the face of interruptions, such adaptability guarantees unbroken quality.
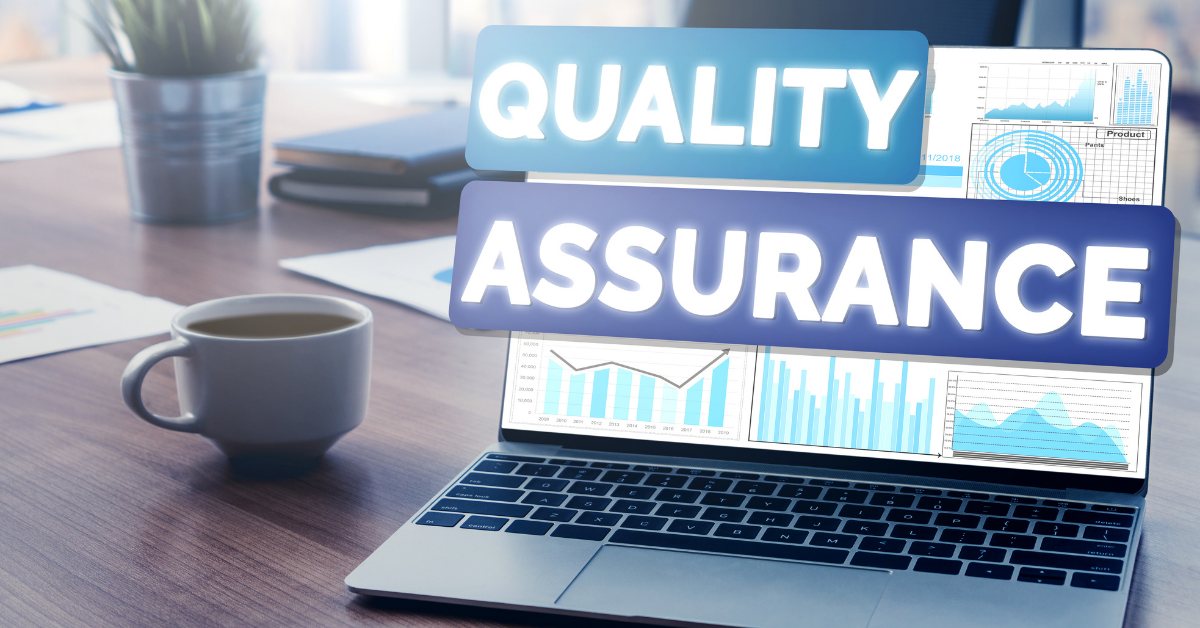
9. Case Study: Artificial Intelligence in Automobile Production
AI-driven quality control is demonstrated at Tesla’s Gigafactories. Cameras and sensors inspect every part of the car, including the paint job and battery cells. Every day, machine learning algorithms examine gigabytes of data to find patterns that might indicate flaws.
AI identified unequal seatbelt tensioners during Model Y manufacturing in 0.5 seconds, compared to 3 minutes for humans to accomplish the same work. This efficiency increased Tesla’s output by 20% while adhering to strict safety regulations.
10. Overcoming Adoption Obstacles for AI
Even though AI has many advantages, manufacturers still have to deal with issues like integration costs and data privacy. Upgrades are necessary because legacy systems sometimes lack connection. However, staggered implementation is possible with modular AI systems.
Employee training is yet another crucial element. Programs for digital literacy and cooperative seminars facilitate the shift. Businesses such as Siemens effectively bridge the skills gap by providing production teams with AI certification training.
The Path Ahead: AI as a Fundamental Aspect of Quality Control
AI is now a need for competitive production, not a luxury. Even small manufacturers may use AI technologies as innovation grows more inexpensive and algorithms become more intelligent. Innovations like quantum machine learning could resolve complex quality problems in a matter of seconds.
Manufacturers who use AI now will dominate the marketplaces of the future. While rivals using antiquated techniques find it difficult to compete, they will create items more quickly, more cheaply, and with greater quality.
Concluding remarks
AI turns quality control into a proactive, data-driven field that can detect minute flaws and anticipate equipment malfunctions. It enables producers to produce excellence on a large scale, delighting stakeholders and consumers alike. AI’s involvement will grow as technology advances, opening up new possibilities for accuracy and productivity.
Explore AI solutions tailored to your production needs to maintain a competitive edge. You may bookmark this page to return to case studies and trends, or you can sign up for our newsletter to receive weekly insights on manufacturing advances. AI-powered quality control is the way of the future.
FAQ:
How does AI improve quality?
- AI improves quality by employing computer vision to quickly identify flaws. It ensures consistent output by predicting faults through the analysis of production data. Accuracy is improved by ongoing learning, which also lowers human error and increases product dependability.
How can AI help quality management?
- By automating inspections and spotting patterns, AI simplifies quality control. It standardizes checks, streamlines procedures, and forecasts equipment breakdowns. Fast fixes are made possible by real-time feedback loops, which reduce waste and guarantee compliance.
What is AI-driven quality control?
- AI-driven quality control uses machine learning to monitor production procedures. It checks goods for defects, modifies operations, and compares data to benchmarks. This method reduces examination times and expenses while guaranteeing accuracy.
What is quality control in manufacturing technology?
- Through testing and inspections, quality control in manufacturing guarantees that goods fulfill requirements. Artificial intelligence (AI) enhances accuracy, avoids flaws, and automates tests. It incorporates data analytics to solve problems proactively.
How can AI be used to improve manufacturing?
- By streamlining processes and anticipating machine failures, AI enhances production. Defect detection is improved, downtime is decreased, and production is personalized. Smarter decisions are made possible by real-time data, which increases productivity and lowers operating expenses.