The Next Development in AI Language Modeling: Qwen 2.5-Max
The introduction of Qwen 2.5-Max marks a significant milestone in the rapidly evolving realm of artificial intelligence. Qwen2.5-Max-Max-Alibaba Cloud’s state-of-the-art large language model (LLM) uses sophisticated architectures and training methods to produce remarkable performance, effectiveness, and adaptability. This blog post examines the technical advancements of Qwen 2.5-Max, contrasts it with rival models, and explains how to include it in your projects while making sure your content complies with Yoast SEO best practices for the greatest possible Google ranking.
Describe QWEN 2.5-Max-Qwen2.5-Max
The newest flagship model in the Qwen series, Qwen2.5-Max, is well-known for its potent language creation and understanding abilities. Qwen2.5-Max is a Mixture-of-Experts (MoE) model that improves performance without making processing more expensive by turning on expert sub-networks only when they are needed during inference. Supervised Fine-Tuning (SFT) and Reinforcement Learning from Human Feedback (RLHF) have been used to further tune this model, which was pre-trained on more than 20 trillion tokens, guaranteeing that its outputs sound natural and are contextually accurate.
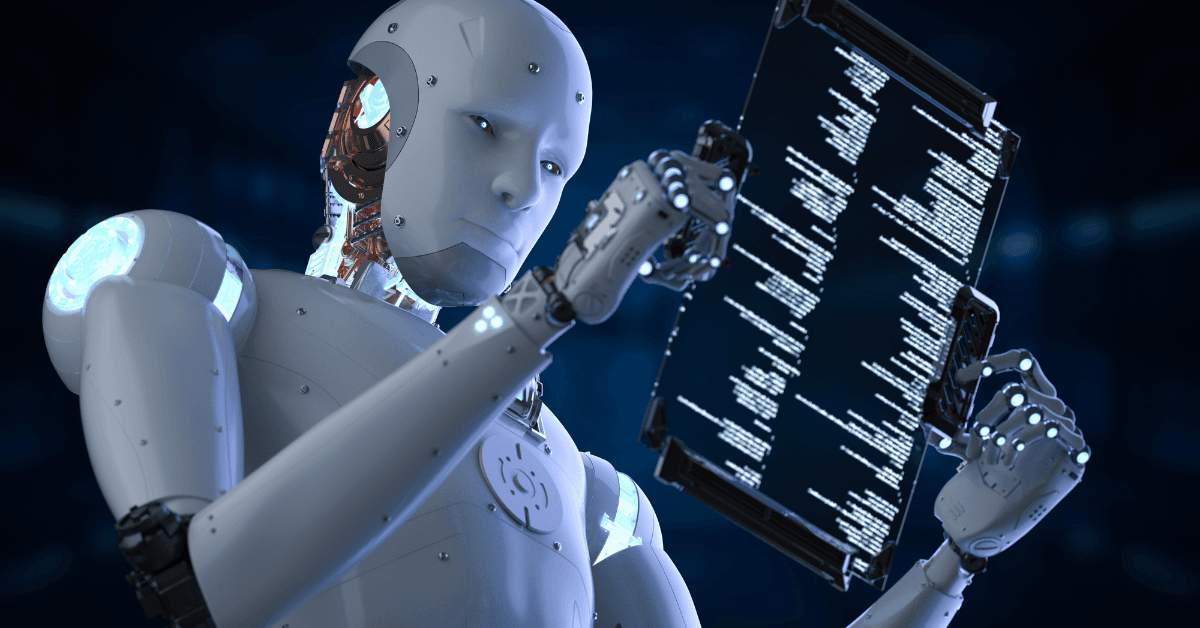
Innovations in Technical Architecture and Mixture-of-Experts (MoE) Design
Qwen2.5-Max’s MoE architecture is its fundamental component. For large-scale applications, traditional dense models may be wasteful since they process each input utilizing every parameter that is available. MoE architectures, on the other hand, separate the model into several expert networks and only activate the most pertinent ones in response to the input query. This selective activation enhances performance and scalability while simultaneously conserving computational resources. Qwen 2.5-Max provides focused and excellent answers on a variety of subjects by simulating a group of experts.
Data Scale and Pretraining-Qwen2.5-Max
Qwen2.5-Max’s intensive pretraining on a dataset with over 20 trillion tokens forms its basis. To put this in perspective, consider that this vast corpus spans a wide range of languages, fields, and styles, from research papers and technical publications to casual talks. The model gains a deep and wide knowledge of English from this enormous amount of training data, which helps it to produce long-form content, manage complicated questions, and even preserve consistent context over lengthy talks.
Adjusting with RLHF and SFT
Fine-tuning is essential for actual performance, even when pretraining creates a strong language foundation. Supervised Fine-Tuning (SFT) is used by Qwen 2.5-Max to direct the model toward generating more accurate and practical results. Furthermore, Reinforcement Learning from Human Feedback (RLHF) aligns the model’s outputs with human preferences. When combined, these methods guarantee that Qwen 2.5-Max not only comprehends user inquiries but also produces dependable, interesting, and context-aware responses.
Benchmarks for performance-Qwen2.5-Max
A rigorous benchmark investigation showed that Qwen 2.5-Max outperformed other cutting-edge models. Some key points:
Arena-Hard and LiveBench: Qwen2.5-Max surpasses DeepSeek-V3 and proprietary systems like GPT-4o in benchmarks that mirror human preferences and capabilities.
Coding and Problem Solving: The model excels at coding and logical reasoning, making it suitable for technical support and code generation.
Mathematics and Structured Data Understanding: The model solves mathematical problems and deciphers tables and JSON well due to its huge training corpus and careful fine-tuning.
Qwen 2.5-Max Comparison with Rival Models
We must evaluate large language models for performance and deployment. Compared to dense models like Claude 3.5-Sonnet and GPT-4o, Qwen2.5-Max has many advantages:
Efficiency: Qwen 2.5-Max’s MoE design lets it infer efficiently without utilizing much computing power.
Qwen 2.5-Max is stable in multi-turn talks and complex thinking issues, according to rigorous testing. It works consistently with long texts and complex instructions.
Developers and enterprises can afford Qwen 2.5-Max because of its low API fee. The pricing is attractive for scaling AI-powered products without incurring significant costs.
Applications and Use Cases
Consumer and commercial settings can employ Qwen 2.5-Max due to its adaptability. Some notable use cases are:
Chatbots & Virtual Assistants: Qwen 2.5-Max’s language understanding and generation skills enable conversational agents that engage and delight.
Content Generation: The model generates logical, contextually rich content for technical manuals, blog posts, and marketing copy.
Coding Help: The model’s coding skills can help developers generate, debug, and solve technical problems.
Analyzing and interpreting data Qwen 2.5-Max can understand and output structured outputs like JSON, making it useful for data processing, summarization, and interpretation.
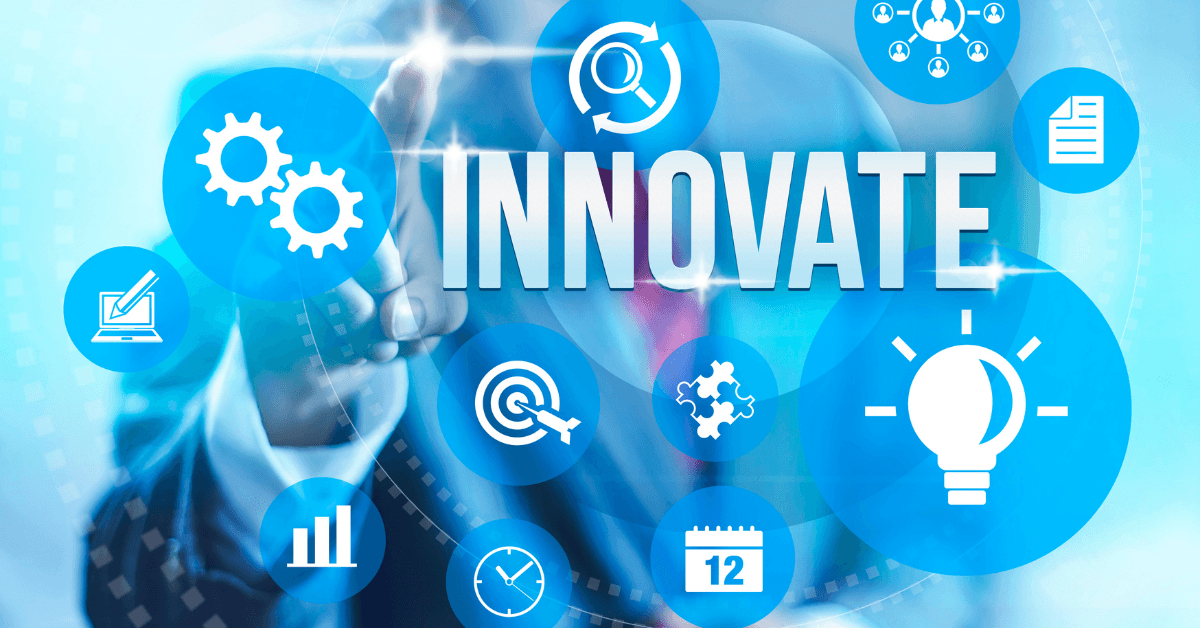
Accessing Qwen 2.5-Max
Qwen 2.5-Max integrates easily into projects. Some typical methods:
The easiest approach to test Qwen 2.5-Max is via the web-based Qwen Chat interface. Create a Google or email account to communicate with the model.
Qwen2.5-Max is available to developers via the Alibaba Cloud Model Studio API. After signing up for Alibaba Cloud and activating Model Studio, you may create an API key to integrate the model into your apps using OpenAI-compatible API calls.
Deployment on Local and Cloud Infrastructure: Installing Qwen 2.5-Max locally or using vLLM, ModelScope, or containerized environments for more extensive integration is covered.
Prospects for the Future and Improvements
As with any other cutting-edge technology, the adventure continues with the release of Qwen 2.5-Max. Alibaba Cloud is constantly looking for methods to improve model reasoning even more, lower latency, and add multilingual support. Even more sophisticated fine-tuning techniques are anticipated in subsequent versions, maybe involving specialized reasoning modules that could further push the limits of AI performance.
Furthermore, it’s critical to maintain constant communication with the development community. Open dialogues, common standards, and cooperative initiatives will keep spurring advancements that enhance Qwen2.5-Max and influence the direction of AI language models in other sectors.
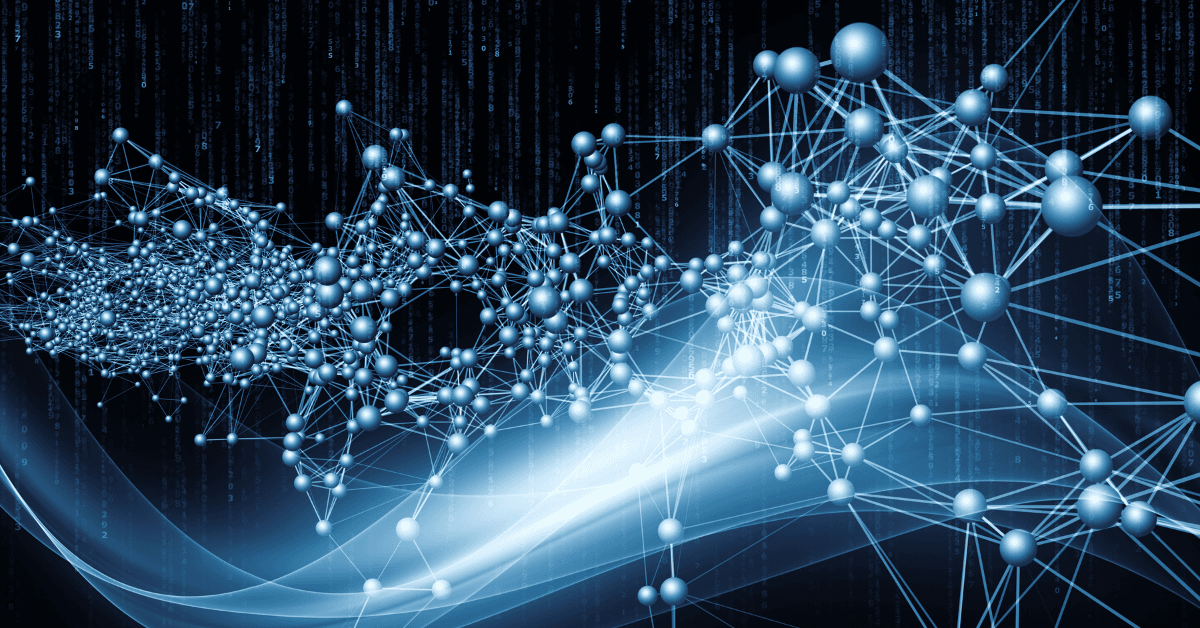
Conclusion and Request for Action
Qwen2.5-Max is a powerful, flexible language model for technical and creative applications. Due to its original MoE design, rigorous pretraining on trillions of tokens, and trustworthy fine-tuning, Qwen2.5-Max could revolutionize artificial intelligence. This architecture is cost-effective and performant for chatbots, content creation, and intelligent data analysis.
Are you prepared to see the newest AI language models in action? Visit the Qwen Chat platform or register for API access through Alibaba Cloud to learn more about Qwen 2.5-Max right now. Boost your projects with state-of-the-art technology and take part in the AI-powered innovation revolution!
Learn about Qwen2.5-Max right now, incorporate it into your apps, and keep up with the latest developments in artificial intelligence. Click here to get started and unlock the future of intelligent automation.
FAQ:
What is Qwen2.5 Max?
- Alibaba Cloud’s huge language model, Qwen2.5 Max, delivers effective, high-quality text creation through the use of a Mixture-of-Experts architecture, pretrained on more than 20 trillion tokens, and refined through supervised and reinforcement learning.
What is the Qwen model?
- Alibaba Cloud created the Qwen family of big language models, which come in a range of sizes and configurations. They facilitate reasoning, sophisticated coding, multilingualism, and challenging visual-language activities.
Is Qwen AI free?
- While premium models like Qwen 2.5 Max require a subscription and are accessible to qualified developers worldwide via Alibaba Cloud’s API platform, Qwen AI is freely available for research usage.